In an age defined by digital transformation, two pivotal domains have emerged as the cornerstones of the tech landscape: data science and cyber security. With the relentless expansion of technology, the need for professionals skilled in these fields has skyrocketed. In this article, we will embark on a comprehensive journey to understand the contrasting yet interconnected realms of data science and cyber security. Join us as we explore their roles, significance, career trajectories, and the skills they demand.
The Essence of Data Science
Data Science in a Nutshell
Data science is the art of extracting valuable insights from vast data sets. It’s a multidisciplinary field that amalgamates statistics, computer science, and domain knowledge. By utilizing cutting-edge techniques like machine learning and artificial intelligence, data scientists uncover patterns, trends, and hidden gems from data oceans.
Role and Responsibilities
Data scientists are the modern-day alchemists, transforming raw data into gold mines of information. They design experiments, analyze data, and build predictive models. For instance, in e-commerce, data scientists predict customer behavior to optimize product recommendations, ultimately boosting sales.
Importance and Applications
The applications of data science are far-reaching. From healthcare predicting disease outbreaks to finance optimizing investment strategies, data science has revolutionized decision-making across industries. In the realm of entertainment, streaming platforms harness user data to personalize content recommendations.
Key Skills
Data science demands a diverse skill set. Proficiency in programming languages such as Python and R is a must. Moreover, data visualization skills using tools like Tableau or Power BI are crucial for presenting complex findings in an understandable manner.
The Essence of Cyber Security
Deciphering Cyber Security
Cyber security, on the other hand, is the safeguarding of digital assets from malicious attacks. It encompasses a wide array of practices, technologies, and processes designed to protect data, systems, and networks from unauthorized access, breaches, and cyber threats.
Role and Responsibilities
Cyber security professionals are the digital guardians. They architect and implement security measures to thwart cybercriminals. Ethical hackers, for instance, emulate cyber attacks to identify vulnerabilities before malicious hackers can exploit them.
Importance and Applications
In an era riddled with data breaches and ransomware attacks, cyber security has taken center stage. From financial institutions securing transactions to governments protecting critical infrastructure, the role of cyber security experts is pivotal.
Key Skills
Proficiency in network protocols and encryption is fundamental for cyber security practitioners. Additionally, they must possess a deep understanding of malware analysis, penetration testing, and risk assessment techniques.
Data Science vs. Cyber Security: The Showdown
Comparison Table
Aspect | Data Science | Cyber Security |
---|---|---|
Focus | Extracting insights from data | Safeguarding digital assets |
Core Skills | Statistics, machine learning, data wrangling | Encryption, risk assessment, penetration testing |
Tools | Python, R, TensorFlow, Tableau | Wireshark, Nessus, Metasploit |
Goals | Informed decision-making | Preventing unauthorized access |
Challenges | Handling noisy data, model selection | Zero-day vulnerabilities, evolving threats |
Career Prospects and Outlook
Data Science Career Trajectory
Data science offers an expansive career landscape. Data scientists, machine learning engineers, and business analysts are in high demand across industries. The rise of big data ensures a steady stream of opportunities for those skilled in extracting meaningful insights.
Cyber Security Career Trajectory
The world’s increasing reliance on digital platforms translates to an ever-growing demand for cyber security professionals. Ethical hackers, security analysts, and information security managers play a pivotal role in safeguarding sensitive information.
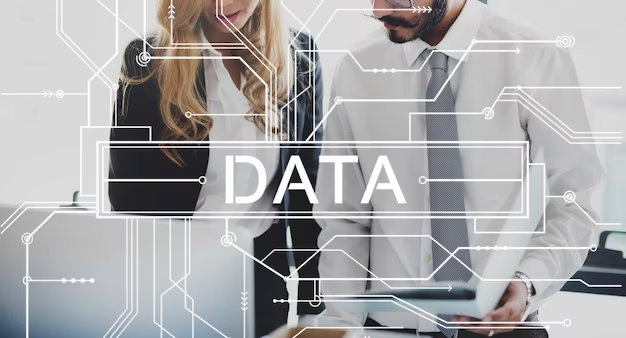
Exploring Data Science Techniques
Unearthing Data Patterns
Data science employs a multitude of techniques to extract valuable insights. Clustering, for instance, groups similar data points together, aiding market segmentation for targeted advertising. Regression analysis helps in predicting future outcomes based on historical data, a crucial tool in finance.
Natural Language Processing (NLP)
NLP is a subfield of data science that focuses on enabling machines to understand and interact with human language. Sentiment analysis gauges public sentiment towards products, brands, or political issues by analyzing social media posts and news articles.
Mastering the Art of Cyber Security
Social Engineering: The Human Factor
While technical skills are vital, understanding human behavior is equally important in cyber security. Social engineering exploits human psychology to gain unauthorized access. Phishing, a common social engineering attack, tricks users into revealing sensitive information.
Incident Response and Recovery
Cyber security is a proactive endeavor. Incident response plans outline strategies for identifying, mitigating, and recovering from security breaches. A well-executed response plan can significantly minimize the damage caused by cyber attacks.
Data Science and Cyber Security Collaboration
Synergy Between the Fields
Data science and cyber security are not mutually exclusive; in fact, they complement each other. Data analysis can help identify patterns that indicate potential security breaches. Moreover, machine learning algorithms can enhance intrusion detection systems by learning to recognize new attack patterns.
Predictive Threat Analysis
By merging data science techniques with cyber security, organizations can predict potential threats and vulnerabilities. This proactive approach empowers companies to fortify their defenses before attackers strike.
The Future: Convergence of Data Security and Cyber Security
Emergence of Secure AI
As artificial intelligence continues to reshape industries, ensuring the security of AI systems becomes paramount. Data security and cyber security professionals will collaborate to build AI models that are both accurate and resilient against adversarial attacks.
Ethical Considerations in AI and Security
The fusion of AI and security raises ethical concerns. Bias in AI algorithms can amplify existing societal inequalities, and the use of AI in cyber attacks can amplify their scale and impact. Striking a balance between innovation and responsibility will be a crucial challenge.
Advanced Data Science Techniques
1. Ensemble Learning Strategies
Ensemble learning combines multiple models to improve predictive accuracy. Techniques like Random Forest and Gradient Boosting create a ‘committee’ of models that collectively make more accurate predictions than individual models.
2. Time Series Analysis
Time series data, such as stock prices or temperature records, requires specialized analysis techniques. ARIMA (AutoRegressive Integrated Moving Average) models and exponential smoothing methods are used to forecast future values based on historical patterns.
3. Dimensionality Reduction
High-dimensional data can be challenging to work with. Techniques like Principal Component Analysis (PCA) and t-SNE (t-distributed Stochastic Neighbor Embedding) reduce data dimensions while preserving essential information, aiding in visualization and analysis.
4. Neural Networks in Data Science
Neural networks, a subset of machine learning, are inspired by the human brain’s neural structure. Deep learning, a type of neural network, excels in tasks like image and speech recognition due to its ability to automatically learn features from data.
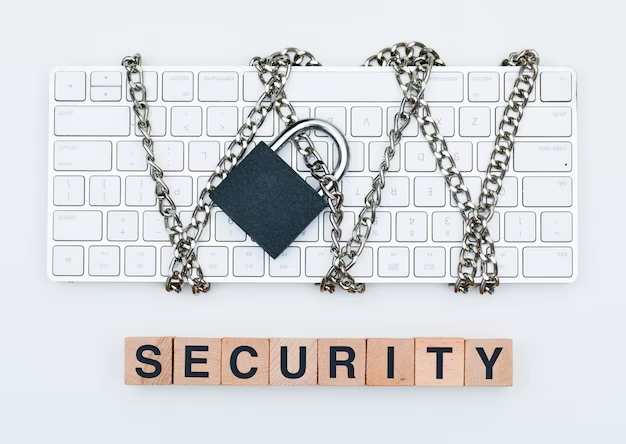
Cutting-Edge Cyber Security Tools
1. Intrusion Detection Systems (IDS)
IDS tools monitor network traffic for suspicious activities or patterns that might indicate an intrusion. Snort and Suricata are popular open-source IDS tools used to detect and prevent cyber attacks.
2. Threat Intelligence Platforms
These platforms gather and analyze vast amounts of data to provide organizations with real-time insights into emerging threats and vulnerabilities. ThreatConnect and Recorded Future are examples of tools that aid in proactively defending against cyber threats.
3. Blockchain in Cyber Security
Blockchain technology ensures secure, tamper-proof transactions by creating a decentralized and transparent record. In cyber security, it’s used for secure data storage, identity verification, and tracking digital assets.
4. AI-Powered Security Analytics
Machine learning algorithms analyze vast amounts of data to detect anomalies, predict potential threats, and automate incident response. IBM QRadar and Splunk are platforms that leverage AI to enhance security analysis.
Collaboration for a Secure Future
1. Data Sharing for Threat Detection
Collaboration between organizations can bolster cyber security efforts. By anonymously sharing threat data and attack patterns, industries can collectively defend against common adversaries.
2. Integrating AI into Security Operations
Data science and cyber security teams collaborate to build AI-driven solutions that enhance security operations. AI-powered tools can automate threat detection, enabling faster response times.
3. Security in the Internet of Things (IoT)
As IoT devices proliferate, ensuring their security is paramount. Data science can be applied to analyze IoT-generated data for potential vulnerabilities and anomalies.
4. Securing Cloud Infrastructure
Data science and cyber security intersect in securing cloud environments. Machine learning algorithms can monitor cloud activities, detect unauthorized access, and predict potential breaches.
Navigating the Interconnected Landscape
1. Dynamic Training and Skillsets
Professionals in both data science and cyber security need to continuously upskill. Evolving technologies demand constant learning, making adaptability a key trait.
2. The Role of Regulations
Data privacy regulations like GDPR and CCPA impact both fields. Data scientists and cyber security experts must ensure compliance while harnessing the power of data.
3. Ethical Considerations
The collection and use of data raise ethical concerns. Both data scientists and cyber security professionals must navigate issues related to user consent, algorithmic bias, and privacy.
4. The Quest for Balance
As the digital landscape evolves, finding the equilibrium between innovation and security remains a challenge. Striking the right balance ensures a resilient and progressive digital ecosystem.
Data Science in Real-World Applications
1. Healthcare Diagnostics
Data science aids in early disease detection through the analysis of medical images and patient records. Machine learning models can identify patterns that might go unnoticed by human clinicians, leading to more accurate diagnoses.
2. Fraud Detection
Financial institutions deploy data science to detect fraudulent activities. Anomalies in transaction patterns are detected by algorithms, preventing unauthorized access to accounts.
3. Recommender Systems
Data science drives personalized recommendations on platforms like Netflix and Amazon. These systems analyze user behavior and preferences to suggest content or products tailored to individual tastes.
4. Climate Modeling
Climate scientists utilize data science to model and predict climate patterns. By analyzing historical climate data, scientists can make informed projections about future climate changes.
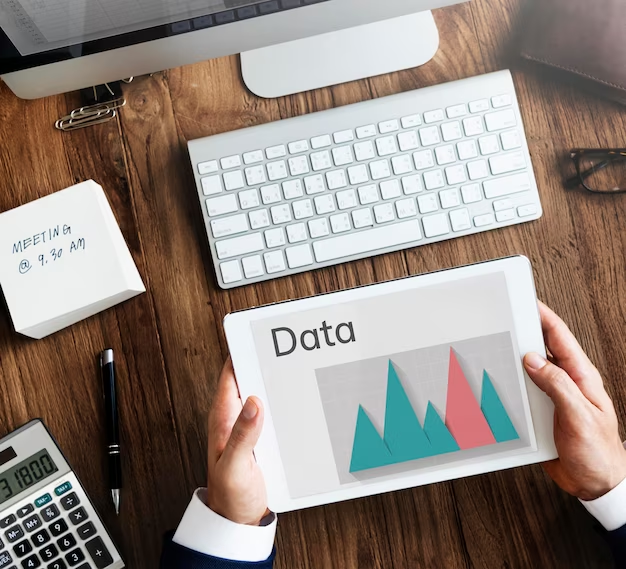
Evolving Trends in Cyber Security
1. AI-Enhanced Threat Detection
Cyber security leverages AI to detect advanced threats that may evade traditional security measures. Machine learning algorithms analyze data to identify unusual patterns indicating potential attacks.
2. Zero Trust Architecture
This paradigm shifts from perimeter-based security to a model where no one is inherently trusted. Every user and device is verified before granting access, minimizing the attack surface.
3. Cloud Security Challenges
As businesses migrate to cloud environments, securing cloud data becomes paramount. Cyber security professionals must address challenges such as data breaches, misconfigurations, and insider threats.
4. Quantum-Safe Encryption
With the advent of quantum computing, traditional encryption methods can be compromised. Cyber security experts are exploring quantum-safe encryption techniques to ensure future-proof data protection.
Bridging the Gap: Data Science and Cyber Security Integration
1. Threat Intelligence Analytics
By integrating data science techniques, threat intelligence can be enhanced. Data analysis can identify correlations in threat data that human analysts might miss.
2. Behavioral Analytics
Data science can help in analyzing user behavior to detect potential security breaches. Patterns deviating from the norm can indicate compromised accounts or insider threats.
3. Security Information and Event Management (SIEM)
SIEM systems collect and analyze security data from various sources. Data science can refine SIEM alerts, reducing false positives and improving threat detection accuracy.
4. Predictive Security Analytics
Data science’s predictive capabilities can aid in anticipating cyber attacks. By analyzing historical attack data and trends, organizations can develop strategies to mitigate future threats.
Preparing for the Future
1. Lifelong Learning
Both data science and cyber security fields demand continuous learning. Professionals should stay updated with the latest technologies and methodologies to remain relevant.
2. Cross-Disciplinary Expertise
The convergence of data science and cyber security calls for professionals with hybrid skill sets. Those who understand both domains can bridge gaps and drive innovation.
3. Ethical and Legal Considerations
As data-driven technologies advance, ethical dilemmas arise. Professionals must navigate issues related to data privacy, consent, and compliance to ensure responsible innovation.
4. Research and Innovation
Data science and cyber security remain dynamic fields with ample scope for research and innovation. Solving complex challenges and pushing boundaries will define the future of these domains.
Emerging Data Privacy Challenges
1. Privacy Regulations
Data privacy laws like GDPR and CCPA dictate how organizations handle personal data. Ensuring compliance is crucial to avoid legal repercussions.
2. De-Identification Techniques
To protect user privacy, data scientists use de-identification techniques that remove or mask personally identifiable information from datasets.
3. Differential Privacy
Differential privacy adds noise to data to protect individual information while still allowing meaningful analysis. It strikes a balance between data utility and privacy.
4. Privacy-Preserving Machine Learning
Researchers are exploring techniques that enable machine learning on encrypted data, ensuring privacy while still deriving insights.
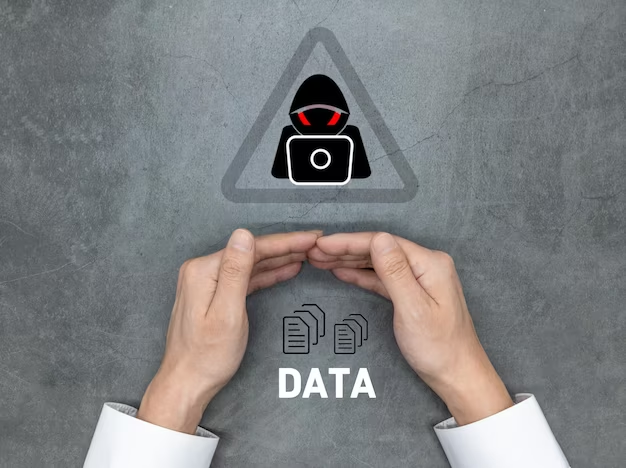
Cyber Security in the Internet of Things (IoT)
1. IoT Vulnerabilities
The proliferation of IoT devices has led to security challenges. Poorly secured devices can be entry points for attackers into larger networks.
2. Device Authentication
Strong authentication methods for IoT devices, such as two-factor authentication and digital certificates, ensure that only authorized devices can connect.
3. Over-the-Air Updates
Regular updates are essential to patch vulnerabilities in IoT devices. Over-the-air updates allow manufacturers to remotely fix security issues.
4. Network Segmentation
Isolating IoT devices on separate networks limits their access to critical systems, reducing the potential impact of a breach.
The Role of Government and Regulation
1. National Cyber Security Strategies
Governments formulate strategies to protect critical infrastructure and combat cyber threats. These strategies often involve partnerships with private sector entities.
2. Cybersecurity Frameworks
Frameworks like NIST Cybersecurity Framework provide guidelines for organizations to assess and improve their cyber security posture.
3. International Collaboration
Cyber threats are borderless, necessitating international cooperation to combat them. Collaborative efforts aim to share threat intelligence and coordinate response.
4. Ethical Use of Data
Governments play a role in shaping regulations that ensure the ethical use of data, addressing concerns related to privacy, consent, and responsible data handling.
Envisioning a Secure and Data-Driven Future
1. Innovations in Data Privacy
Technological advancements will likely bring new methods to safeguard individual privacy while still enabling effective data analysis.
2. AI-Powered Security
AI’s role in cyber security will expand further, with advanced machine learning models becoming a mainstay in threat detection and prevention.
3. Ethical AI and Security
The integration of ethics into AI and security practices will ensure responsible and unbiased decision-making in both domains.
4. Continuous Evolution
Both data science and cyber security will continue to evolve, creating a dynamic landscape of innovation, challenges, and opportunities.
Conclusion
In the dynamic landscape of technology, data science and cyber security emerge as pillars of innovation and protection. While data science uncovers the hidden truths within data, cyber security shields our digital assets from malicious forces. As you traverse these domains, remember that your choice between data science and cyber security depends on your passion for unraveling data mysteries or defending the digital realm. Embrace the path that resonates with you, for both avenues offer boundless opportunities to make a lasting impact in the digital age.
FAQs
Absolutely! Many skills, such as analytical thinking and programming, are transferrable between the two fields, making such transitions feasible.
Industries like healthcare, e-commerce, finance, and marketing heavily leverage data science for decision-making and optimization.
AI enhances cyber security by automating threat detection, analyzing patterns in real-time, and identifying anomalies that might go unnoticed by human analysts.
While a degree in computer science or statistics is common, individuals from diverse backgrounds can enter data science by acquiring relevant skills through online courses and practical projects.
Yes, both fields raise ethical questions related to data privacy, algorithm bias, and responsible AI deployment.